Observability has evolved beyond traditional monitoring, integrating AI, automation, and security. Initially, monitoring focused on collecting logs and metrics separately, often leading to silos and limited visibility. The rise of distributed systems and microservices has increased the need for organizations to implement complete observability frameworks.
Cloud-native environments are driving the need for advanced observability solutions as organizations are increasingly pressured to innovate within these environments. This growing observability trend highlights the need for comprehensive solutions to manage complexity across various technology stacks.
For a deeper dive into how observability has evolved, explore our breakdown of Observability Trends in 2023 and Observability Trends in 2024. Looking back at 2024, modern observability has focused on handling the complexity of cloud systems. Key trends like automation, AI, and better data management have helped teams monitor performance and fix problems faster.
Organizations can use artificial intelligence insights, together with predictive analytics and cost-optimized strategies to improve their successful management of complex infrastructure systems. This new approach improves system resilience while minimizing downtime and simplifying troubleshooting operations.
1. Cut Costs through Data Management
As part of the growing observability trend, companies are adopting smarter data collection methods to reduce unnecessary data and lower storage costs. By sampling key traces, storing only important logs, and moving less critical data to lower-cost storage, businesses can cut costs by 60-80%. These strategies help preserve valuable insights on slow response times, error trends, and system performance while reducing spending and improving query efficiency.
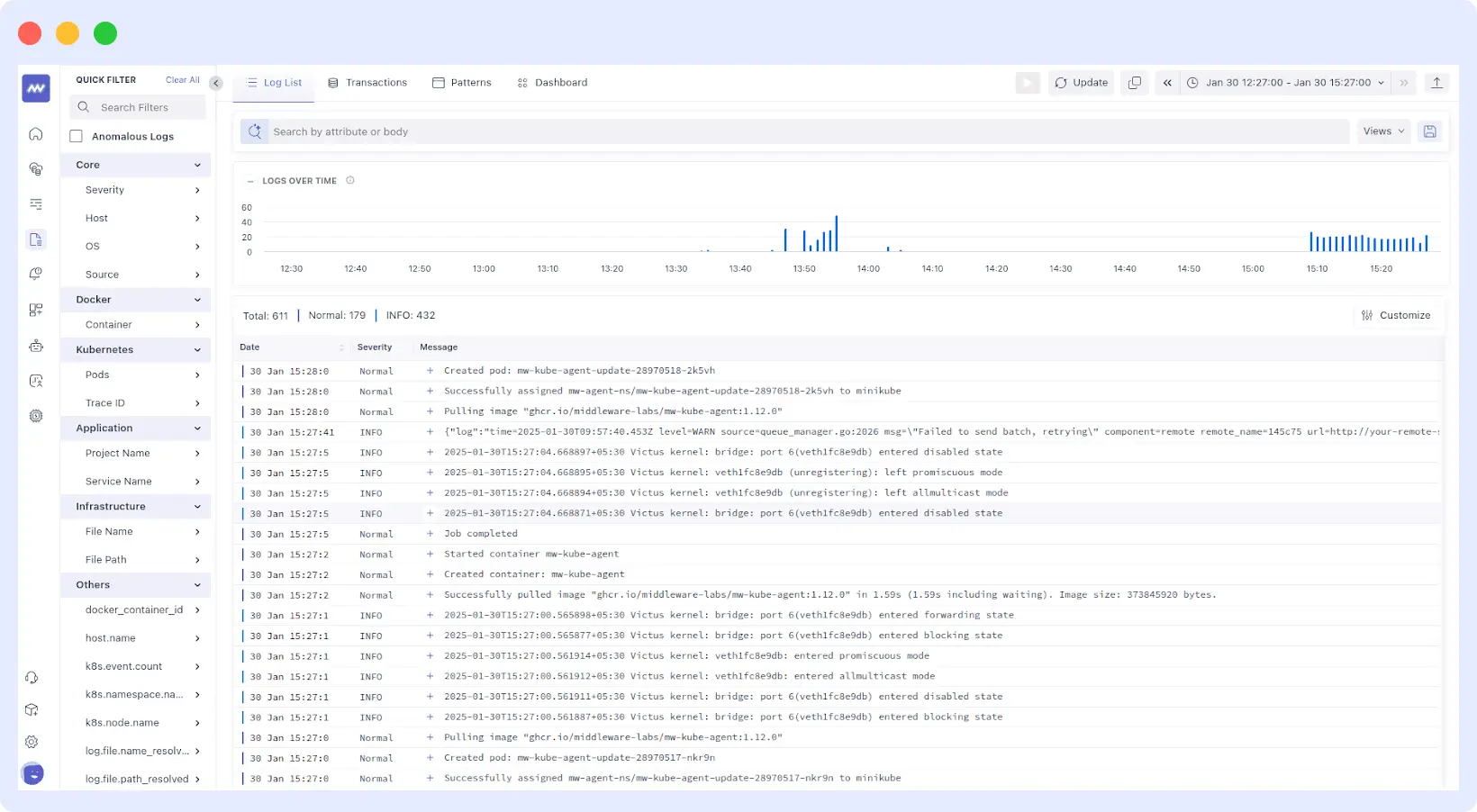
The above dashboard displays log data from the containers. It categorizes logs by severity (Normal, INFO, WARN) and timestamps, providing easy filtering options for containers, infrastructure, and applications.
The top graph visualizes log activity over time, helping with monitoring and troubleshooting events such as agent updates, container startups, and network changes. Additionally, the dashboard offers tagging capabilities, allowing you to filter and retrieve only the logs you need, ensuring you stay focused on the most relevant data.
“Organizations have realized that nearly 70% of collected observability data is unnecessary, leading to inflated costs,”
explains Laduram Vishnoi, Founder and CEO of Middleware.io.
By optimizing data management, companies reduce costs without compromising visibility into system health.
2. AI-Driven Predictive Operations
In the evolving observability trend, traditional monitoring detects issues only after disruptions occur, while AI systems go further by identifying patterns in performance data and predicting potential failures, like resource bottlenecks or memory leaks. This proactive approach helps prevent downtime, optimize resources, and create more resilient and efficient systems.
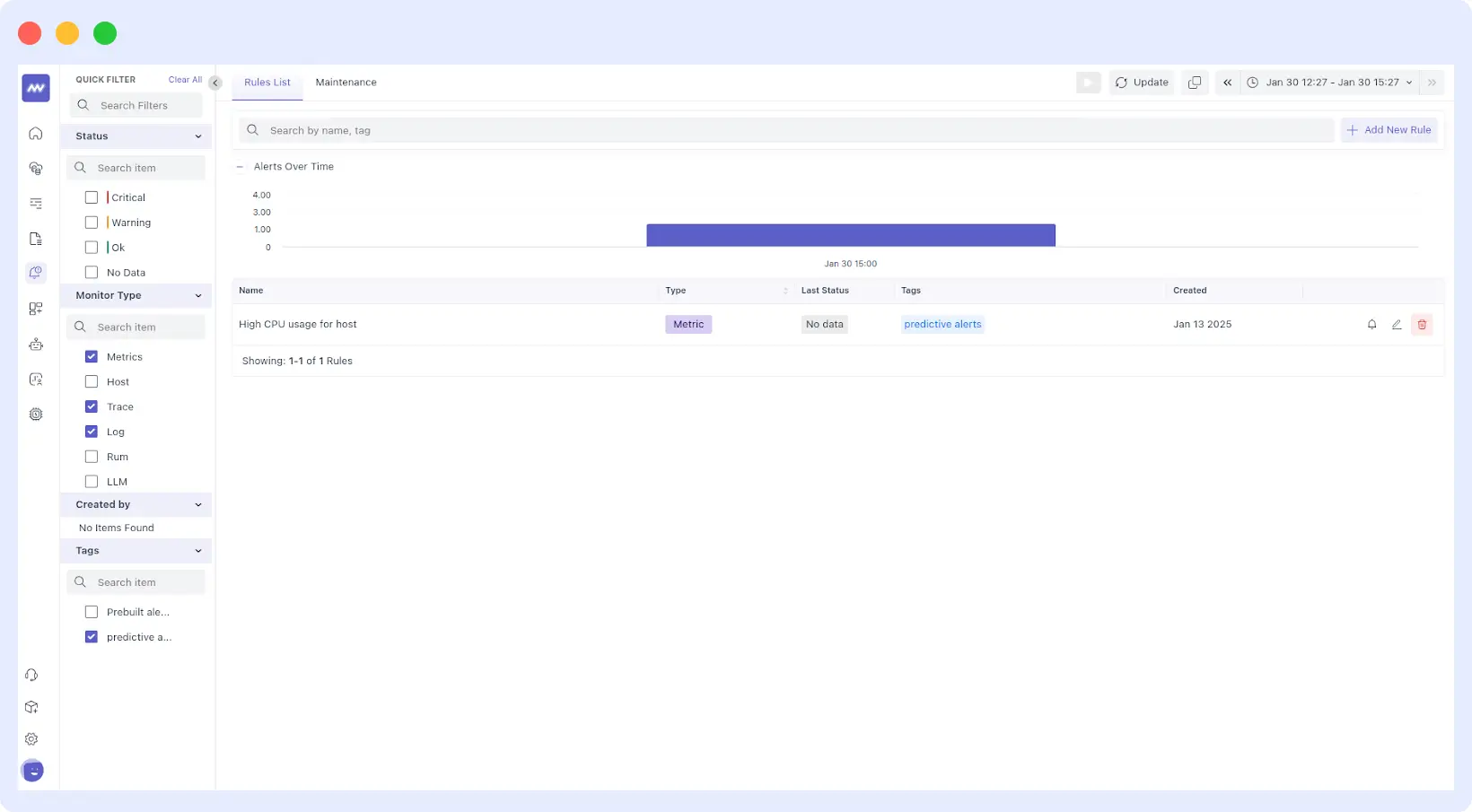
The dashboard displays a predictive alerting system that monitors system health. It shows a high CPU usage alert for a host, triggered by the health of containers and categorized under predictive alerts, showcasing AI-driven monitoring in action.
The graph at the top visualizes the alert activity over time, enabling teams to detect early warning signs and take proactive measures before system failures occur.
“There’s a growing demand for observability systems that can predict service outages, capacity issues, and performance degradation before they occur. This proactive approach allows organizations to address risks and manage resources effectively, ensuring minimal impact on end-users. Predictive alerting, powered by AI, is set to become an industry standard, enhancing reliability and reducing unplanned downtime.“
Sam Suthar, Founding Director, Middleware
3. Integration of AI-Driven Intelligence
With AI, observability transforms systems that not only detect problems but also provide AI-driven insights as corrective solutions before issues escalate. AI-powered analytics tool processes real-time big data to identify anomalies, forecast potential system failures, and perform automated remediation activities, preventing users from encountering problems.
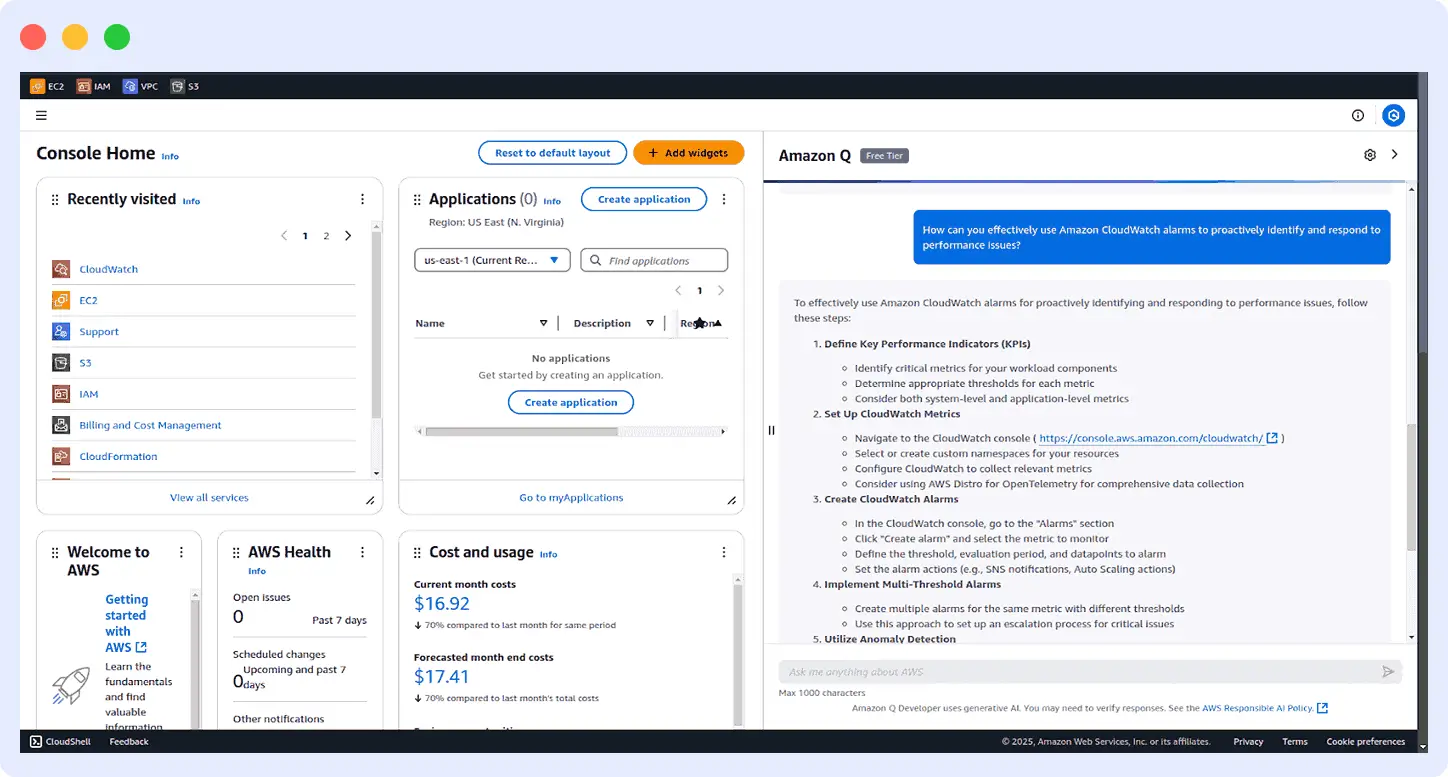
For example, Amazon Q, an AI-driven observability platform, monitors large datasets from AWS services to detect irregular patterns and predict system failures based on historical trends. The AI then automatically triggers actions such as scaling resources or reconfiguring settings to resolve issues before they impact the system. This proactive approach ensures high availability and performance by optimizing infrastructure health in real time, preventing issues like server overload, resource bottlenecks, and application latency.
“Artificial intelligence is being leveraged to improve observability by predicting potential issues before they occur.“
As Laduram Vishnoi notes,
This AI-driven approach reduces downtime, improves system reliability, and accelerates troubleshooting, allowing teams to maintain stability and provide uninterrupted services.
4. AI-Powered Full-Stack Observability
As part of the observability trend, AI upgrades observability by correlating logs, traces, and metrics to detect anomalies across multiple data sources. Unlike traditional tools that analyze data separately, AI looks at everything together, providing deeper insights. It quickly identifies problems by analyzing both real-time and past data, allowing teams to act before issues escalate, reducing downtime, and speeding up problem resolution.
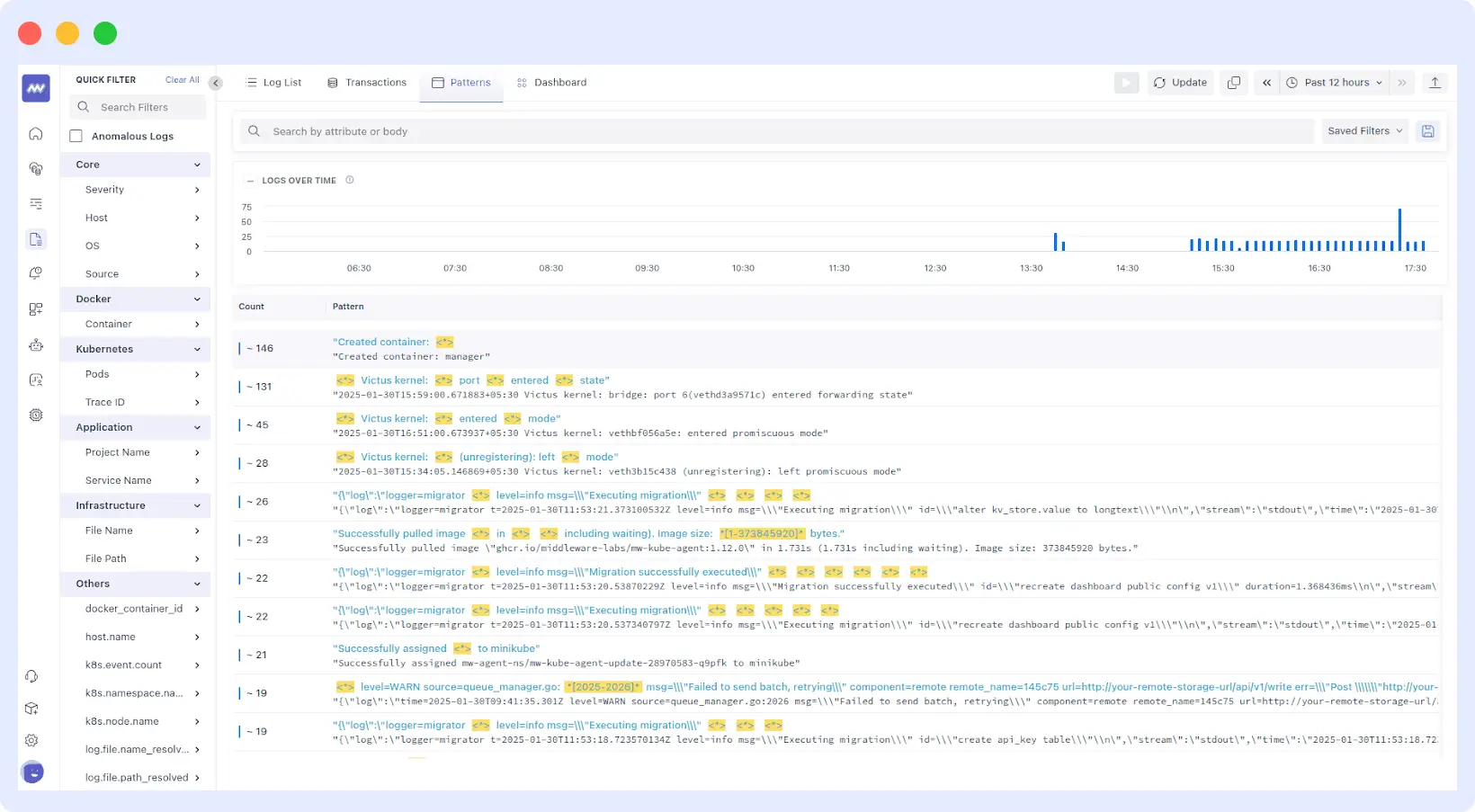
The above dashboard shows how the logs and metrics from containers from the applications are in the same workspace. AI analyzes this data to spot trends and predict issues before they affect the system. By connecting data from different sources, AI helps teams identify and fix problems faster, improving system stability and performance. This approach enables better decision-making and ensures smooth operations.
5. Shift to Flexible Pricing Models
As organizations seek better cost control, observability providers should offer more flexible pricing options. Many now adopt pay-as-you-go models, allowing companies to scale their observability tools without committing to high upfront costs.
“Newer observability providers are offering pay-as-you-go models, allowing companies to choose between long-term commitments and shorter-term arrangements,”
says Laduram Vishnoi.
This model ensures that companies only pay for what they use, optimizing observability costs without compromising functionality.
For example, AWS CloudWatch charges based on the volume of logs processed, such as paying per million log events, which can quickly add up in large-scale environments. Flexible pricing models like pay-as-you-go ensure that organizations can control costs while still benefiting from comprehensive observability tailored to their usage needs.
6. Observability Automation Improves Troubleshooting Capabilities
As organizations scale, managing vast datasets becomes increasingly complex, making traditional root cause analysis slow and prone to errors. AI-powered automation simplifies this process, guiding users through debugging steps and delivering faster insights, reducing operational overhead and human error.
Generation Esports, an EdTech company that uses esports to boost student engagement, faced difficulties in monitoring and fixing problems in their microservices as they expanded. After transitioning from Datadog to Middleware’s observability platform, they cut their observability costs by 75% and resolved infrastructure issues more than 75% faster than before. This automation improved the reliability of their systems and made operations more efficient, giving their engineering team more time to focus on important projects instead of constant troubleshooting.
“Automating observability processes aims to expedite root cause analysis. Currently, sifting through vast data sets to find the root cause of issues is complex and time-consuming. AI-driven automation improves this by providing guided debugging steps, reducing human error, and accelerating issue resolution. As automation capabilities expand, the industry anticipates significant reductions in MTTR, transforming the user experience and operational efficiency.”
Sam Suthar, Founding Director, Middleware
7. AI Observability for AI Workloads
As AI adoption increases, observability platforms are evolving to monitor AI workloads effectively. These platforms track tasks like model training and inference while also optimizing resource use, such as GPU and monitor infrastructure, ensuring cost efficiency.
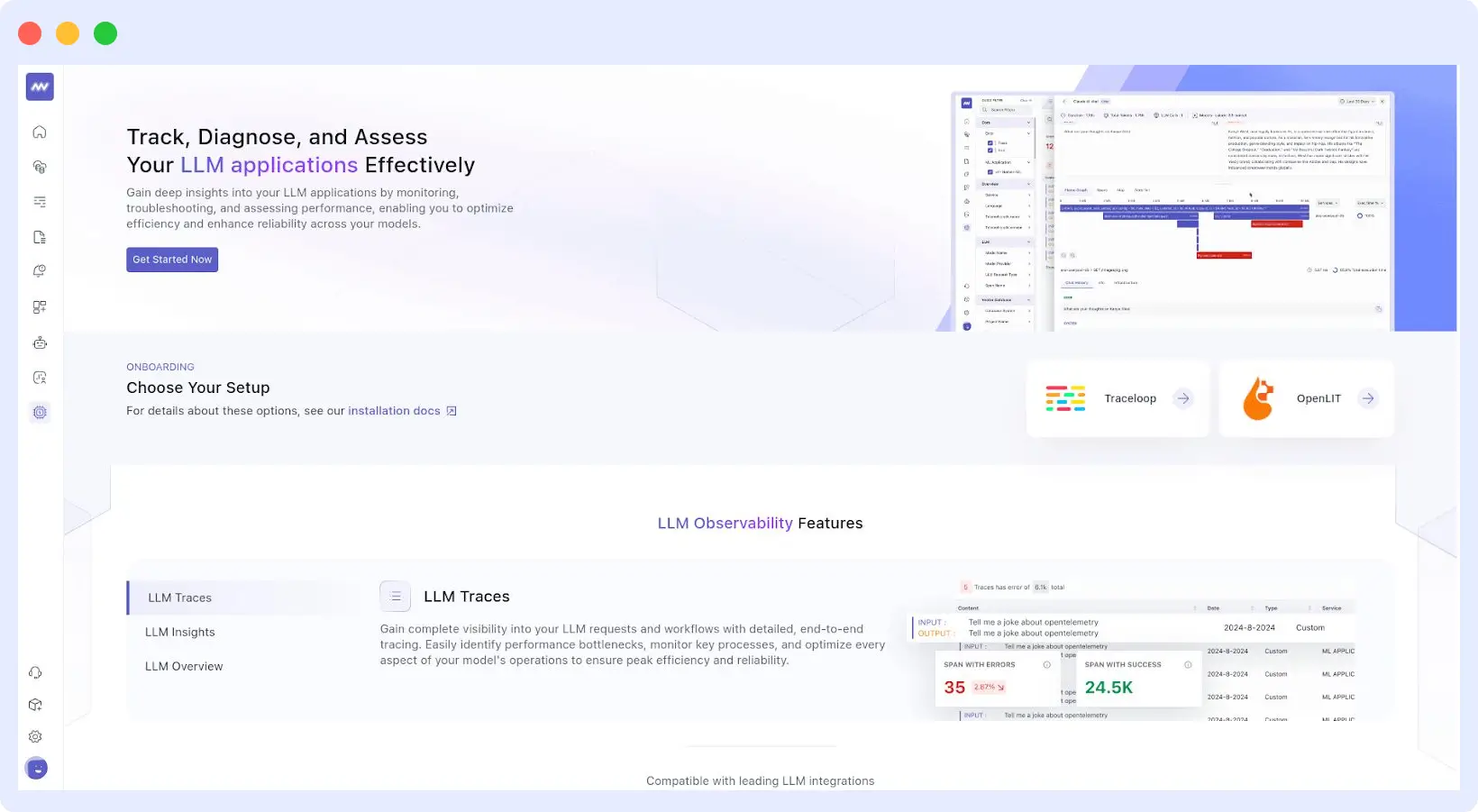
AI observability tools focus on API usage, model performance, GPU utilization, and data processing. They provide insights into API performance, monitor model accuracy and latency, and track GPU usage to ensure resources are being used efficiently. This helps detect inefficiencies and prevent issues like underutilized resources or slow models.
These tools also help manage costs by identifying over-provisioned or underutilized resources. With features like GPU monitoring and performance tracking, AI observability ensures smooth operations while reducing costs associated with token mismanagement and excessive data processing. The image above illustrates how these insights are displayed in a unified dashboard, enabling efficient management of AI workloads.
8. OpenTelemetry Becomes the Default Standard
As part of the observability trend, OpenTelemetry simplifies observability in multi-cloud systems by providing a unified, open-source framework that integrates with popular monitoring tools like Datadog, Prometheus, and AWS CloudWatch. Its multi-cloud observability feature makes monitoring and debugging applications across different cloud environments easier. OpenTelemetry works seamlessly with all major observability tools, consolidating everything into one system for more efficient management.
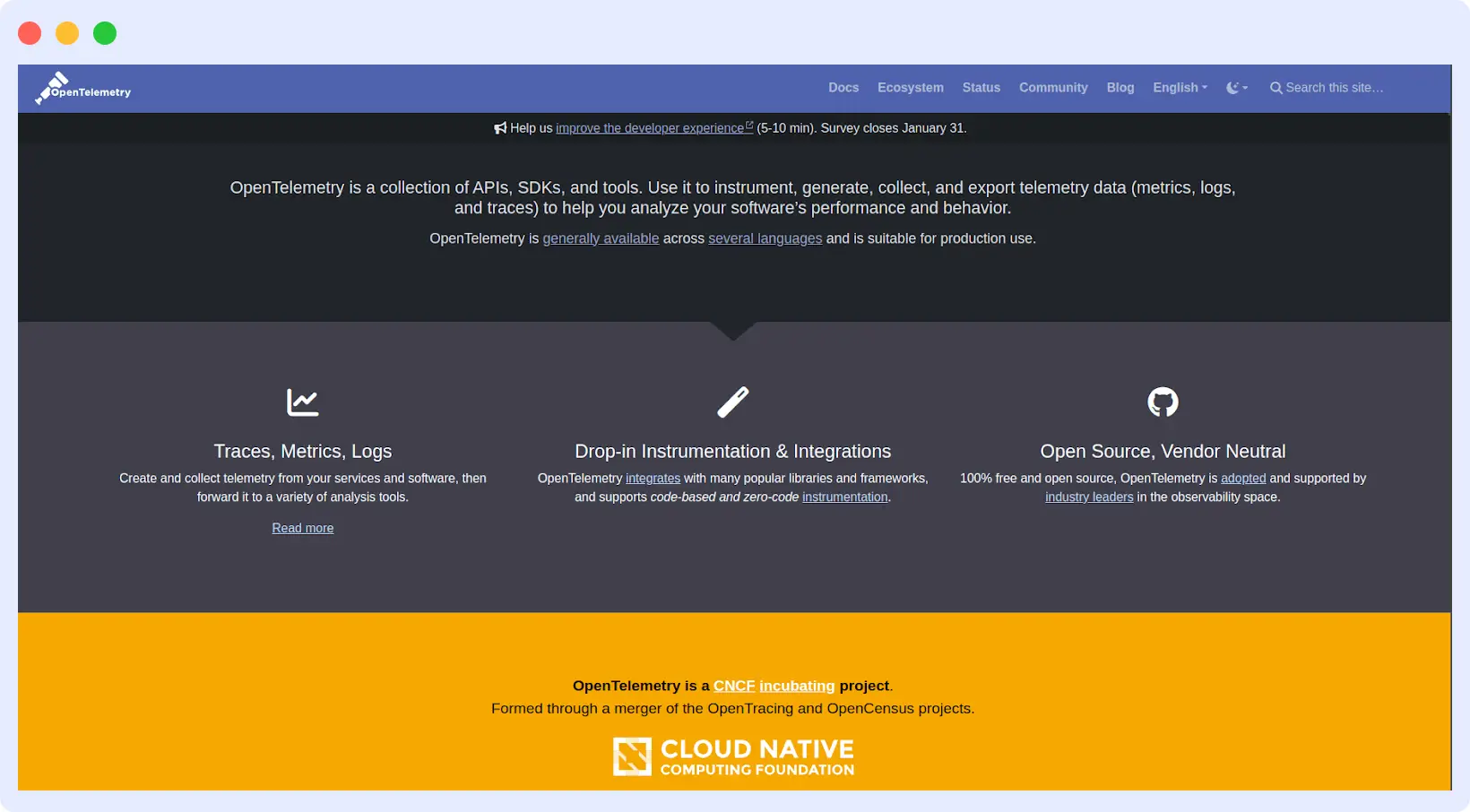
Due to its vendor-neutrality, OpenTelemetry gives businesses greater flexibility by preventing them from being restricted to a single provider. As more businesses adopt OpenTelemetry, they can monitor all of their systems consistently and affordably without being dependent on any one supplier. This guarantees improved control over performance and dependability while simplifying the management of complex cloud configurations.
9. Security-Integrated Observability Becomes a Priority
In line with the observability trend, businesses are integrating security measures into their observability tools as cyber threats become more advanced. Tools like Datadog, Splunk, and Middleware combine security data with performance indicators to detect potential vulnerabilities, such as unusual traffic patterns or unauthorized access attempts. These systems monitor anomalous activity, such as sudden traffic surges or suspicious logins, and notify teams quickly, enabling a fast response to potential security issues.
Netflix monitors logs, metrics, and traces across its services by incorporating security into its observability framework through the use of tools such as OpenTelemetry. According to their Tech Blog, this technology looks for irregularities like sudden increases in traffic or unsuccessful login attempts, which could be signs of security risks like DDoS attacks or illegal access. Combining security and operational data allows Netflix to recognize threats and take quick action. The system automatically initiates warnings and takes action, like banning questionable IP addresses, if it detects suspicious activity.
This method shortens detection and response times, ensuring smooth service and strengthening security for millions of users.
10. Observability Goes Multi-Dimensional
Organizations are including cost, compliance, and security data in their observability frameworks in addition to metrics for performance. Developers can get an in-depth understanding of their infrastructure by combining these various elements.
Under this one framework, teams from DevOps, SecOps, and FinOps can collaborate and make better, more informed decisions. FinOps controls expenses, SecOps keeps an eye on security, and DevOps concentrates on performance, making sure everything works together for improved management.
AWS combines performance, security, and cost metrics in one platform using CloudWatch and Security Hub. This helps teams spot inefficiencies, detect security risks, and monitor performance, all in real-time. By merging these data points, organizations can make smarter decisions, improve workflows, and reduce costs.
Conclusion
In 2025, observability will transform organizations through AI integration, automation, and cost-effective frameworks, improving system resilience, security, and operational efficiency. Data management optimization cuts down unnecessary costs, and vendor-agnostic frameworks deliver improved adaptability. The deployment of AI-based intelligent systems minimizes equipment downtime and enables advanced predictive maintenance.
Flexible cost structures enable organizations worldwide to better manage their observability expenses. Security-integrated observability strengthens cyber resilience, and OpenTelemetry simplifies the task of monitoring multi-cloud environments. Organization-wide implementation of these developments enables the creation of resilient observability frameworks that fulfill current infrastructure demands at scale while remaining affordable.
FAQ
What is the market trend in observability?
Observability solutions utilize AI automation and predictive analytics to improve operational efficiency. Today’s businesses adopt AI-driven observability alongside OpenTelemetry and security-centric monitoring features to ensure reliable performance and reduce operating costs. The market increasingly seeks adaptable pricing structures and data reduction strategies to manage observability expenses more effectively and manageably.
What are the 5 pillars of data observability?
Data observability is built on five key pillars that ensure reliable and accurate data management.
Quality ensures that data remains accurate, consistent, and complete, maintaining integrity across workflows and supporting reliable decision-making.
Lineage maps the flow of data across systems, identifying dependencies and ensuring transparency, which helps prevent disruptions and errors in data processing.
Quality ensures that data remains accurate, consistent, and complete, maintaining integrity across workflows and supporting reliable decision-making.
Freshness guarantees that data is up to date and available when needed, preventing delays in decision-making caused by outdated information.
Volume monitors data for missing, duplicated, or abnormal patterns, helping to detect inconsistencies and ensuring the right amount of data is processed.
Schema tracks structural changes in data, such as unexpected modifications to tables or columns, which could potentially break data pipelines.
What are the four golden signals of observability?
The four golden signals of observability provide a structured approach to monitoring system performance.
Errors: Monitors failed requests and system failures, enabling teams to detect and resolve issues quickly.
Saturation: Assesses resource usage and capacity limits, ensuring the system operates efficiently without being overwhelmed.
Latency: Measures the response time of a system or service, ensuring minimal delays for users.
Traffic: Tracks the volume of incoming requests and overall system load, helping identify potential bottlenecks.
What is modern observability?
Modern observability is the practice of monitoring and understanding system health and performance in complex, cloud-native environments. It goes beyond traditional monitoring by integrating metrics, logs, and traces to provide deeper insights into system behavior. This approach enables proactive issue detection, faster troubleshooting, and enhanced operational efficiency, often with AI and automation to optimize performance and resolve issues.