Summary: Observability is a hot topic for organizations managing complex IT environments, but there’s a lot of confusion about what it really means, why it’s necessary, and what it can actually deliver. This article breaks down what observability is, what it promises, and how it can help organizations achieve their goals.
Today, most developers struggle to comprehend the inner workings and interactions between various components of their IT environments, which are crowded with microservices and other distributed systems.
And without the ability to aggregate, correlate and analyze the performance data of these applications alongside their hardware and network, maintaining and troubleshooting them becomes a huge challenge.
This is where the control theory spin-off, a.k.a observability, comes into the picture.
So, let’s deep dive into what observability is, how it works and its various benefits to businesses.
What is Observability?
In simple words, observability is the ability to assess a system’s current state based on the data it produces. It provides a comprehensive understanding of a distributed system by looking at all the input data.
It is a set of practices that helps developers understand the details of distributed systems throughout their developmental and operational lifecycle.
Typically, observability helps developers gain end-to-end real-time visibility of their distributed infrastructure. It enables them to monitor key performance indicators and metrics, troubleshoot and debug applications and networks, detect anomalies, identify patterns or trends, and address issues before they impact the bottom line.
This way, developers can create a resilient and scalable IT infrastructure that works in tandem with continuous integration and continuous delivery (CI/CD) pipelines while ensuring optimal health and performance.
It’s safe to say that observability has evolved from being a buzzword to an essential requirement for data-driven companies.
History of Observability
Though observability became a business catalyst in the last decade, it boasts a surprisingly long history that dates back to the 17th century, originating from an idea that had nothing to do with software development. Back in the day, engineers like Christiaan Huygens used control theory to understand complex systems like windmills. By taking note of external outputs, such as blade speed, they could determine the internal state and grinding efficiency.
Observability was not until the 1960s that it carved its niche in computer science. Even though computers at the time were relatively basic compared to today’s systems, they were considered complex. As computers became more intricate, the need to monitor their health and performance became abundantly clear. The rise of the Internet two decades later added to the increasing complexity, paving the way for the development of siloed monitoring tools that focused primarily on servers, network traffic, and basic application health.
In the early 2000s, application performance monitoring gained steam, with vendors like AppDynamics and Dynatrace leading the way with tools that provided deep insights into application behavior and helped developers identify performance bottlenecks.
The cloud revolution of the 2010s further transformed observability. While cloud providers like IBM, AWS, and Microsoft offered built-in tools that allowed developers to monitor various aspects of the cloud, companies like Datadog delivered unified platforms to monitor the holy trinity of observability: metrics, logs, and traces. In an effort to expedite adoption and ensure accessibility, open-source solutions like Prometheus also entered the market.
“Each decade has brought a sea change in how observability is expected to function. The last three decades have seen transformation after transformation — from on-premise to cloud to cloud-native. With each generation has come new problems to solve, opening the door for new companies to form.”
Laduram Vishnoi, Founder and CEO of Middleware.
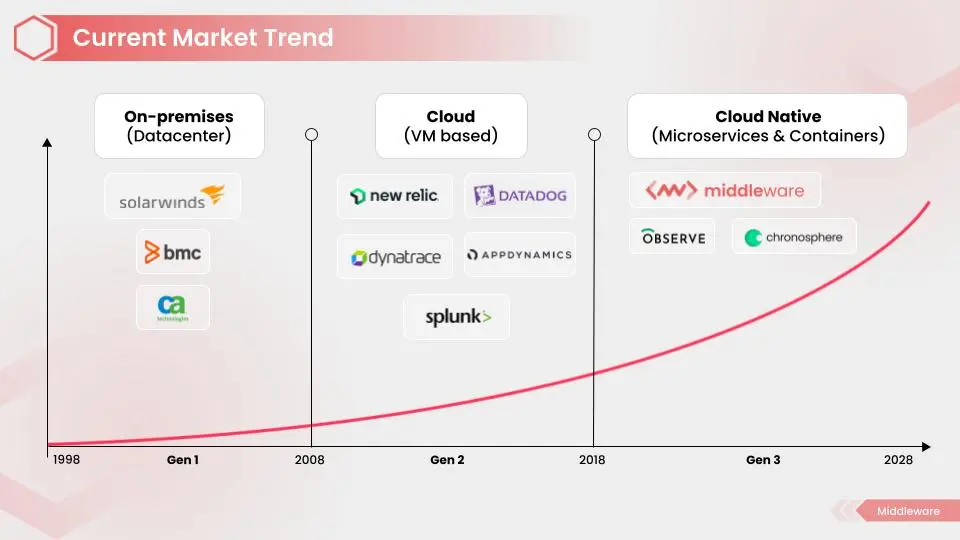
The best observability examples
Today, observability is no longer a niche practice. Here are seven companies known for their cutting-edge observability practices:
- Netflix
Apart from being a global leader in streaming services, Netflix is a champion of microservices architecture. They have built a powerful internal platform dubbed “Chaos Monkey” that intentionally injects failures to identify weaknesses and ensure service resilience. Additionally, they use open-source tools like Prometheus for metrics collection and Grafana for data visualization.
- Facebook by Meta
With its massive user base and ever-evolving applications, Facebook prioritizes performance and observability. The social networking platform uses a combination of custom-built tools and open-source solutions like Prometheus and Zipkin for distributed tracing.
- Uber
Uber relies heavily on microservices and real-time data analysis. Their observability approach focuses on tools like Datadog and Jaeger for tracing requests across microservices, pinpointing issues within individual services without impacting the entire system. Additionally, they monitor data pipelines using tools like Prometheus to ensure data quality for real-time decision-making.
- Airbnb
Much like Uber, Airbnb utilizes observability for managing bookings and guest experiences. They use tools like Datadog and Honeycomb for comprehensive log aggregation and analysis, allowing them to identify issues and optimize guest experiences.
- Spotify
With a vast music library and millions of users, Spotify utilizes observability for performance optimization and a seamless streaming experience. They use a combination of open-source tools like Prometheus and Grafana for infrastructure monitoring, alongside custom-built solutions for application-specific requirements.
- Slack
Slack uses observability to identify and resolve issues quickly, minimizing downtime for real-time communication. They use tools like Datadog for real-time monitoring of their infrastructure and applications, allowing them to detect and address issues proactively.
How does observability work?
Observability operates on three pillars: logs, metrics, and traces. By collecting and analyzing these elements, you can bridge the gap between understanding ‘what’ is happening within your cloud infrastructure or applications and ‘why’ it’s happening.
With this insight, engineers can quickly spot and resolve problems in real-time. While methods may differ across platforms, these telemetry data points remain constant.
Logs
Logs are records of each individual event that happens within an application during a particular period, with a timestamp to indicate when the event occurred. They help reveal unusual behaviors of components in a microservices architecture.
- Plain text: Common and unstructured.
- Structured: Formatted in JSON.
- Binary: Used for replication, recovery, and system journaling.
Cloud-native components emit these log types, leading to potential noise. Observability transforms this data into actionable information.
Start collecting and monitoring logs from any environment in 60 seconds. Get started!
Metrics
Metrics are numerical values describing service or component behavior over time. They include timestamps, names, and values, providing easy query ability and storage optimization.
Metrics offer a comprehensive overview of system health and performance across your infrastructure.
However, metrics have limitations. Though they indicate breaches, they do not shed light on underlying causes.
Traces
Traces complement logs and metrics by tracing a request’s lifecycle in a distributed system.
They help analyze request flows and operations encoded with microservices data, identify services causing issues, ensure quick resolutions, and suggest areas for improvement.
Unified observability
Successful observability stems from integrating logs, metrics, and traces into a holistic solution. Rather than employing separate tools, unifying these pillars helps developers gain a better understanding of issues and their root causes.
As per recent studies, companies with unified telemetry data can expect a faster Mean time to detect (MTTD) and Mean time to respond (MTTR) and fewer high-business-impact outages than those with siloed data.
How is observability different from monitoring?
Cloud monitoring solutions employ dashboards to exhibit performance indicators for IT teams to identify and resolve issues. However, they merely point out performance issues or help developers gain visibility into what’s happening without any solid explanation as to why it’s happening.
As such, monitoring tools must get better at overseeing complex cloud-native applications and containerized setups that are prone to security threats.
In contrast, observability uses telemetry data such as logs, traces, and metrics across your infrastructure. Such platforms provide useful information about the system’s health at the first signs of an error, alerting DevOps engineers about potential problems before they become serious.
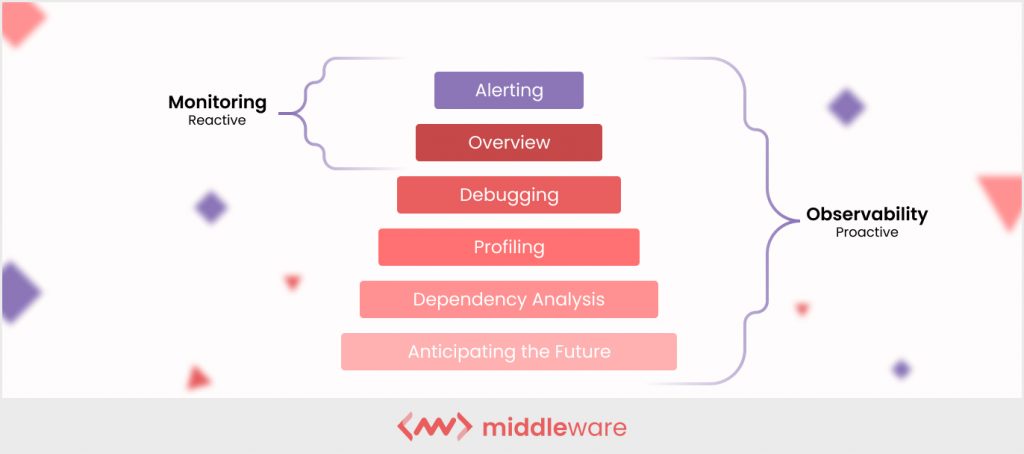
Observability grants access to data encompassing system speed, connectivity, downtime, bottlenecks, and more. This equips teams to curtail response times and ensure optimal system performance.
As per recent reports, nearly 64% of organizations using observability tools have experienced mean time to resolve (MTTR) improvements of 25% or more.
Read more about Observability vs. Monitoring.
Why is observability important for business?
In the last decade, the emergence of cloud computing and microservices has made applications more complex, distributed, and dynamic. In fact, over 90% of large enterprises have adopted a multi-cloud infrastructure.
While the shift to scalable systems has benefited businesses, monitoring and managing them has become very challenging. These challenges are:
- Companies understand that the tools they previously relied on don’t fit the job.
- Legacy monitoring systems lack visibility, create siloed environments, and hinder process management and automation efforts.
- Vendor lock-in—switching from one old vendor to a new one was tedious. But using observability-based vendor-agnostic data formats, you can easily import data.
- Legacy platform overcharges costs; sometimes, it costs more than cloud hosting.
It is no surprise that DevOps and SRE teams are turning to observability to understand system behavior better and improve troubleshooting and overall performance. In fact, the increasing dependency on observability platforms has the potential to bolster the market by 2028 with USD 4.1 billion.
The benefits of observability
According to the Observability Forecast 2023, organizations are reaping a wide range of benefits from observability practices:
Improved system uptime and reliability
Developers want their applications to be as available and reliable as possible. But this is easier said than done in the real world, as distributed systems are extremely challenging to implement.
Observability tools offer developers real-time insights into system health and behavior, empowering them to pinpoint and resolve issues before they can cause an outage. This subsequently leads to higher uptime and makes the overall system robust.
Of all the benefits observability can offer, improved system uptime and reliability are considered to be the most important.
Increases operational efficiency
With real-time insights into system performance and behavior, better operational efficiency is an absolute given. If done right, developers can automate repetitive tasks and optimize resource consumption and operations.
Improves security vulnerability management
Beyond DevOps, observability tools are extremely beneficial for security and DevSecOps teams as they allow them to track and analyze security breaches or vulnerabilities in real time and resolve them. This ensures a secure application environment.
Enhances real-user experience
Observability tools like Middleware offer a range of features that specifically target end-user experience.
For example, with capabilities like real user monitoring (RUM), developers can gain comprehensive user journey visibility for web/mobile applications. This enables them to identify and troubleshoot issues concerning front-end performance and user actions, correlate those issues, and make data-driven decisions to address them.
Understand user journey with session replays. Get started for free.
Improves developer productivity
“Developers spend nearly 50% of their time and effort on debugging. Observability tools have the potential to bring that down to 10%, allowing developers to focus on more critical areas.”
Laduram Vishnoi, Founder and CEO of Middleware.
Wondering how Middleware helps developers move over debugging? Check out our exclusive feature on YourStory.
Observability tools don’t just offer comprehensive visibility into distributed applications; they render actionable insights that developers can actually use to identify and fix bugs, optimize code, and enhance overall productivity.
“I don’t want my developers to stay up all night to try to fix issues. That’s a waste of everyone’s time. Middleware helped us become faster. It saves at least one hour of my time every day, which I can dedicate to something else.”
Akshat Gupta, Trademarkia.
The real advantage of using observability?
These were just the tip of the iceberg. Companies using full-stack observability have seen several other advantages:
- Nearly 35.7 % experienced MTTR and MTTD improvements.
- Almost half the companies using full-stack observability were able to lower their downtime costs to less than $250k per hour.
- More than 50% of companies were able to address outages in 30 minutes or less.
Additionally, companies with full-stack observability or mature observability practices have gained high ROIs. In fact, 71% of organizations see observability as a key enabler to achieving core business objectives. As of 2024, the median annual ROI for observability stands at 100%, with an average return of $500,000.
How can Observability benefit Devops and engineers?
Observability is so much more data collection. Access to logs, metrics, and traces marks just the beginning. True observability comes alive when telemetry data improves end-user experience and business outcomes.
Open-source solutions like OpenTelemetry set standards for cloud-native application observability, providing a holistic understanding of application health across diverse environments.
Real-user monitoring offers real-time insight into user experiences by detailing request journeys, including interactions with various services. This monitoring, whether synthetic or recorded sessions, helps keep an eye on APIs, third-party services, browser errors, user demographics, and application performance.
With the ability to visualize system health and request journeys, IT, DevSecOps, and SRE teams can quickly troubleshoot potential issues and recover from failures.
Throwing AI into the mix makes everything better.
AI can enhance observability by using telemetry data to improve end-user experiences and business outcomes.
Blending AIOps (the practice of using AI and Machine Learning to enhance and automate IT operations) and Observability can optimize real user monitoring and automate the analysis of vast data streams, allowing teams to maximize their overall efficiency to a great extent. Other benefits include:
- Automating incident detection and resolution by analyzing historical data, identifying patterns, and predicting potential issues.
- Correlating unrelated or non-specific data points to pinpoint the underlying cause of an error.
Some observability tools powered by AI can automatically identify performance bottlenecks and suggest improvements, helping developers improve overall system performance and UX.
Top 6 observability best practices
There is no doubt that observability offers immense value. However, it’s important to understand that most available tools lack business context.
On top of that, several organizations look at technology and business as two separate disciplines, hindering their overall ability to maximize their use of observability. The situation highlights the need for a defined set of best practices.
- Unified telemetry data: Consolidate logs, metrics, and traces into centralized hubs for a comprehensive overview of system performance.
- Metrics relevance: Identify and monitor important metrics that are aligned with organizational goals.
- Alert configuration: Set benchmarks for those metrics and automate alerts to ensure quick issue identification and resolution.
- AI and machine learning: Leverage machine learning algorithms to detect anomalies and predict potential problems.
- Cross-functional collaboration: Foster collaboration among development, operational, and other business units to ensure transparency and overall performance.
- Continuous enhancement: Regularly assess and improve observability strategies to align with evolving business needs and emerging technologies.
Read more about observability best practices.
Finding the right observability tool
Selecting the right observability platform can be a tad bit difficult. You must consider capabilities, data volume, transparency, corporate goals, and cost.
Here are some points worth considering:
User-friendly interface
Dashboards present system health and errors, aiding comprehension at various system levels. A user-friendly solution is crucial for engaging stakeholders and integrating smoothly into existing workflows.
Real-time data
Accessing real-time data is vital for effective decision-making, as outdated data complicates actions. Utilizing current event-handling methods and APIs ensures accurate insights.
Open-source compatibility
Prioritize observability tools using open-source agents like OpenTelemetry. These agents reduce resource consumption, enhance security, and simplify configuration compared to in-house solutions.
Easy deployment
Choose an observability platform that can quickly be deployed without stopping daily activities.
Integration-ready across tech stacks
The tools must be compatible with your technology stack, including frameworks, languages, containers, and messaging systems.
Clear business value
Benchmark observability tools against key performance indicators (KPIs) such as deployment time, system stability, and customer satisfaction.
AI-powered capabilities
AI-driven observability helps reduce routine tasks, allowing engineers to focus on analysis and prediction.
Top 5 observability platforms
You can easily employ these observability platforms once you have a clear idea of your organizational goals and use cases. Here are the leading five options:
Middleware
Middleware is a cloud-based observability platform that breaks down data and insight barriers between containers. It can quickly identify the root causes of problems, detect both infrastructure and application issues in real-time, and provide solutions.
Furthermore, Middleware unites metrics, logs, and traces in a single dashboard to help solve problems quickly, reducing downtime and improving user experience.
“Middleware has proven to be a more cost-effective and user-friendly alternative to New Relic, enabling us to capture comprehensive telemetry across our platform. This improved our operational efficiency, service delivery, and accelerated incident root cause analysis.”
John D’Emic, Co-Founder and CTO at Revenium.
Splunk
Splunk is an advanced analytics platform powered by machine learning for predictive real-time performance monitoring and IT management. It excels in event detection, response, and resolution.
Datadog
Datadog is designed to help IT, development, and operations teams gain insights from a variety of applications, tools, and services. This cloud monitoring solution provides useful information to companies of all sizes and sectors.
Dynatrace
Dynatrace provides both cloud-based and on-premises solutions with AI-assisted predictive alerts and self-learning APM. It is easy to use and offers various products that render monthly reports about application performance and service-level agreements.
Observe, Inc.
Observe is a SaaS tool that provides visibility into system performance. It provides a dashboard that displays the most important application issues and overall system health. It is highly scalable and uses open-source agents to gather and process data, simplifying the setup process quickly.
Observability challenges in 2024
Here’s an interesting question: if observability provides so many advantages, then what’s stopping organizations from going all in?
Cost: In 2023, nearly 80% of companies experienced pricing or billing issues with an observability vendor.
Data overload: The sheer volume, speed, and diversity of data and alerts can lead to valuable information surrounded by noise. This fosters alert fatigue and can increase costs.
Team segregation: Teams in infrastructure, development, operations, and business often work in silos. This can lead to communication gaps and prevent the flow of information within the organization.
Causation clarity: Pinpointing actions, features, applications, and experiences that drive business impact is hard. Companies need to connect correlations to causations regardless of how great the observability platform is.
The future of observability
As 2024 unfolds, the future of observability holds exciting possibilities.
In the days to come, the industry will see a major shift, moving away from legacy monitoring to practices that are built for digital environments.
Full-stack observability tops this list, with nearly 82% of companies gearing up to adopt 17 capabilities through 2026. The idea of tapping natural language and Large Language Models (LLMs) to build more user-friendly interfaces is also gaining steam.
Furthermore, industry players are upping the ante by tapping into AI to offer unified systems of records, end-to-end visibility, and high scalability.
They promise to democratize observability, deliver real-time insights into operations, reduce downtime, improve user experiences, and ensure customer satisfaction.
Middleware is leading this change with its full-stack observability solutions that can unify telemetry data into a single location and deliver actionable insights in real time.
This helps organizations better manage multi-cloud environments and ensure seamless migrations. Such a comprehensive approach to observability can help companies make the most of multi-cloud infrastructures.
Schedule a free demo with one of our experts today!
FAQs
What is observability?
Observability entails gauging a system’s present condition through the data it generates, including logs, metrics, and traces. Observability involves deducing internal states by examining output over a defined period. This is achieved by leveraging telemetry data from instrumented endpoints and services within distributed systems.
Why is observability important?
Observability is essential because it provides greater control and complete visibility over complex distributed systems. Simple systems are easier to manage because they have fewer moving parts.
However, in complex distributed systems, monitoring is necessary for CPU, logs, traces, memory, databases, and networking conditions. This monitoring helps in understanding these systems and applying appropriate solutions to problems.
What are the three pillars of observability?
The 3 pillars of observability: Logs, metrics and traces.
- Logs: Logs provide essential insights into raw system information, helping to determine the occurrences within your database. An event log is a time-stamped, unalterable record of distinct events over a specific period.
- Metrics: Metrics are numerical representations of data that can reveal the overall behavior of a service or component over time. Metrics include properties such as name, value, label, and timestamp, which convey information about service level agreements (SLAs), service level objectives (SLOs), and service level indicators (SLIs).
- Traces: Traces illustrate the complete path of a request or action through a distributed system’s nodes. Traces aid in profiling and monitoring systems, particularly containerized applications, serverless setups, and microservices architectures.
How do I implement observability?
Your systems and applications require proper tools to gather the necessary telemetry data to achieve observability. By developing your own tools, you can utilize open-source software or a commercial observability solution to create an observable system. Typically, implementing observability involves four key components: logs, traces, metrics, and events.
What are the best observability platforms?
- Middleware
- Splunk
- Datadog
- Dynatrace
- Observe, Inc