Imagine this: your production environment is behaving erratically, with requests timing out and error messages everywhere. With a traditional logging solution, identifying the root cause—especially in distributed apps—usually requires a long and laborious process. OpenTelemetry logging addresses this problem by easing and speeding up troubleshooting and debugging.
Read on to understand what OpenTelemetry Logging is, how to collect OpenTelemetry logs and how to leverage Middleware to tackle the challenges of log management in distributed systems.
What is OpenTelemetry?
OpenTelemetry is an open-source, vendor-neutral observability framework that provides a standard, unified approach to capturing telemetry data in modern applications. It offers a set of APIs, instrumentation libraries, SDKs and integrations for collecting telemetry data, including metrics, traces, and logs.
OpenTelemetry is built and designed around three core components: instrumentation, collection and exporting. The instrumentation component allows you to add code to your app for telemetry collection.
Once your app is instrumented, the OpenTelemetry Collector gathers telemetry from various sources in your stack at runtime and processes it for further analysis. OpenTelemetry then exports the collected telemetry to various backends, such as observability platforms, logging systems and monitoring tools.
What are OpenTelemetry Logs?
OpenTelemetry Logs are time-stamped text records of events and activities with metadata. Any data—such as events or spans—that is not part of a distributed trace or a metric in OpenTelemetry is a type of or attached to a log.
Logs provide detailed information about app health—including errors, warnings, and other important events. They facilitate debugging and enable you to make informed decisions for app improvement. With OpenTelemetry, you can integrate alerting systems that notify you when specific preconfigured log patterns or keyword triggers are detected.
OpenTelemetry logs are collected using a different approach compared to metrics and traces. For metrics and traces, OpenTelemetry provides a new API and its implementation in various languages.
But logs have a rich history of collection and usage; popular programming languages traditionally incorporated logging capabilities or libraries into their framework. As such, OpenTelemetry supports existing logging libraries while improving on their capabilities and abstracting their integrability challenges.
In essence, with OpenTelemetry’s “Logs Bridge API,” you can collect log data by incorporating existing logging libraries—regardless of the programming language used— into your stack.
Additionally, OpenTelemetry connects logs to traces and metrics to provide richer telemetry for easier troubleshooting. It also captures span events that provide context to allow you to easily interpret logs. Let’s briefly compare OpenTelemetry logs and spans.
Logs vs. Span Events
Logs are records of critical events, errors and warnings, and the time they occur within an app. They provide comprehensive records of an app’s behavior during its execution and facilitate real-time monitoring.
Span events, on the other hand, capture the context of operations within an app, including timing and causal relationships between different components. OpenTelemetry correlates logs with other observability data via execution time, execution context and resource context.
Span IDs, when included in LogRecords, provide the resources for correlating logs with traces that correspond to the same execution context.
A span typically includes metadata, a start time, an end time, and a set of log events associated with that span. This makes spans highly valuable for correlating logs of individual events from various services in distributed systems.
Why is Data Correlation Important?
Data correlation is important for several reasons, including the following.
Easy Root Cause Analysis
Correlating logs, metrics, and traces allows for effective root cause analysis. Aside from the root cause, correlated data also makes it easy to identify and understand the sequence of events leading to an incident and accurately pinpoint the exact components or services that are contributing to the problem. This facilitates efficient issue remediation and reduces the chances of the issue reoccurring.
Performance Optimization
By analyzing correlated metrics, logs, and traces, you can identify bottlenecks, inefficiencies, or resource-intensive processes. This information enables you to make data-driven decisions to optimize app performance, fine-tune configurations and allocate resources more effectively.
Holistic View
By combining data from different sources such as apps, hosts (e.g VMs and containers) and host components, correlation enables you to understand the interdependencies between various components, services, and infrastructure.
You will also gain insights into the end-to-end flow of requests, understand system-wide impacts during incidents and visualize the relationships between metrics and logs of various components.
OpenTelemetry’s Logs Data Model
This is the model and semantic conventions that allow logs to be represented from various sources, such as application log files, machine-generated events, system logs, etc.
OpenTelemetry’s logs data model seeks to achieve the following goals:
- To unify the status of logs—what a log record is, what data needs to be recorded, transferred, stored and interpreted by a logging system.
- To easily map legacy log formats to this data model.
- To allow for easy translation of heterogeneous data formats to and from this data model.
- To ensure log formats converted to and from this data model are semantically meaningful.
- To enable efficient representation of the data model in concrete implementations that require data storage or transmission.
- To represent 3 core types of logs—first-party apps, system formats (e.g. Syslog) and third-party apps (e.g. Apache log file).
Types of Logs Gathered by OTel
Let’s take a brief look at the 3 types of logs and events that the OpenTelemetry Logs Data Model aims to represent.
- System Formats: These are logs generated by the OS. You generally have limited control over their format unless they are generated by an app that can be modified.
- Third-party Application Logs: These are logs generated by third-party apps. You may be able to customize their format, but you typically have limited control over them.
- First-party Application Logs: These are logs generated by your app. You have more control over how they are generated and what information is included. You can also modify the source code of the app to make changes.
Log Records
A log record provides details of an app event and contains two kinds of fields—which include descriptions of the logs character. The fields are discussed below.
Named Top-level Fields
These are fields of specific type and meaning. They include mandatory or regularly occurring fields in both legacy and upcoming log formats (e.g., Timestamps and TraceIds, respectively). The semantics of top-level fields must be identical across all known log and event formats. It must also be easily and unambiguously convertible to the OpenTelemetry logs data model.
Resource and Attributes Fields
Also called arbitrary key-value pairs, these fields are stored as “map<string, any>” and offer flexibility for log representation. They allow you to define custom fields and values in log messages using standardized or arbitrary semantic conventions. They capture information specific to the application’s needs, such as user IDs, request IDs and error codes.
The fields are described in the table below.
Field Name | Description |
Timestamp | This represents the time the event occurred, measured by the source time. |
ObservedTimestamp | This shows the time the event was observed by the collection system. |
Trace Context Fields | These include the TraceId, SpanId and TraceFlags, and are useful in data correlation. |
SeverityText | This is also known as log level, which are TRACE, DEBUG, INFO, WARN, ERROR, FATAL. |
SeverityNumber | This is the numerical value of severity; includes 1-4 for TRACE, 5-8 for DEBUG, 9-12 for INFO, 13-16 for WARN, 17-20 for ERROR, and 21-24 for FATAL. |
Body | This is the main message of the log record. It can be a human-readable string message describing the event in a free form. |
Resource | This describes the source of the log. It can contain information about the instrumented app or the infrastructure on which the app runs. |
InstrumentationScope | This describes the scope that emitted the log. It is often represented in a tuple of strings. |
Attributes | This contains additional information about the event. Unlike the Resource field, which is fixed for a particular source, Attributes can vary for each occurrence of the event coming from the same source. |
These fields are usually represented in a typical log record as exemplified below.
OpenTelemetry Log Record: An Example
This is what an OTel’s Log record might look like, following the logs data model and in JSON format.
"Timestamp" : "1634630600000",
"ObservedTimestamp" : "1634630601000",
"TraceId" : "xyz7890",
"SpanId" : "ijkl4321",
"SeverityText" : "INFO",
"SeverityNumber" : "6",
"Body" : "A successful request has been processed.",
"Resource" : {
"service.name" : "web-backend",
"host.name" : "web-server-2"
},
"InstrumentationScope": {
"Name" : "JavaLogger",
"Version": "1.0.0"
},
"Attributes" : {
"http.method": "POST",
"http.status_code": "200"
}
So, how do we collect this data?
Methods of Log Data Collection
Whether you are instrumenting a system, first-party app or third-party app, OpenTelemetry offers two approaches to data collection. We’ll discuss them below.
Via File or Stdout Logs
This is a method where logs are written to an intermediary medium (e.g. file or stdout). An important advantage of this method is that it minimizes the need for changes in the way logs are produced and where they are written by the application.
The approach requires the ability to read file logs and handle them correctly, even when log rotation is used. The approach may also optionally require the ability to parse the logs and convert them into more structured formats using various types of parsers.
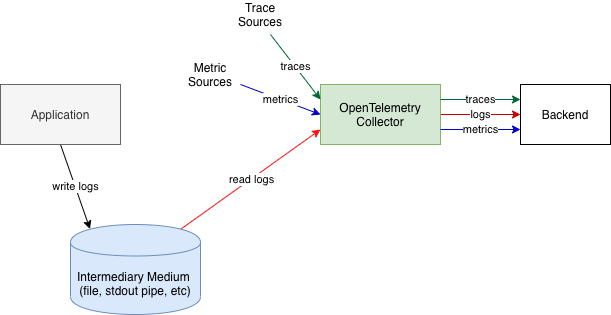
To do this, OpenTelemetry recommends using the Collector or (if it is unable to) other log collection agents (e.g., FluentBit). Parsers can be configured to handle custom log formats or common ones—such as CSV, Common Log Format, LTSV, Key/Value Pair format, and JSON.
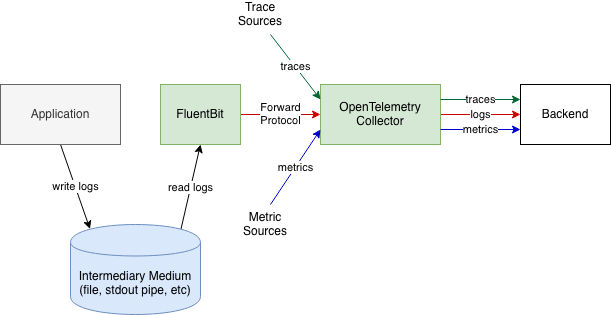
An important drawback of using the intermediary medium is that it requires file reading and parsing, which can be difficult, time-consuming, and unreliable if the output format is poorly defined.
Direct to Collector
This approach involves modifying the application to output logs via a network protocol, such as OTLP. This can be achieved conveniently by providing add-ons or extensions for commonly used logging libraries. The add-ons send logs over the selected network protocol. This requires you to make minimal localized changes to your app code, typically focused on updating the logging target.
Once logs are collected, the Collector enriches them with resource context, similar to how it is done for third-party apps. This enrichment ensures that the logs have comprehensive correlation information across all context dimensions.
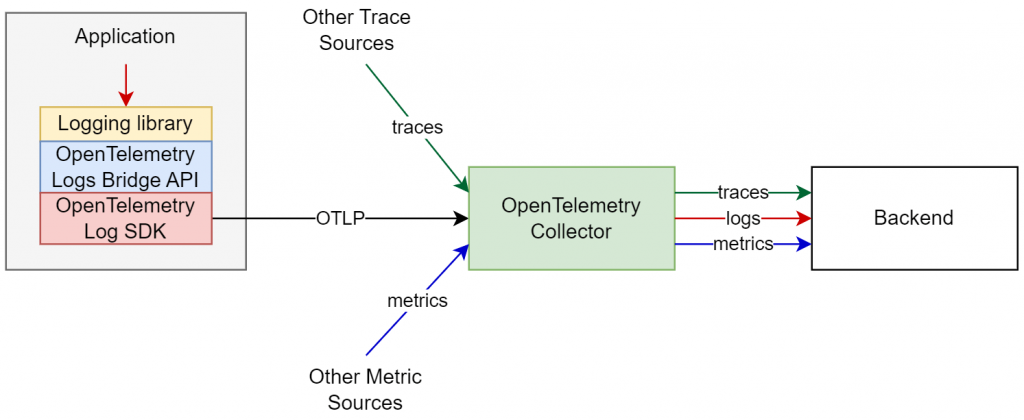
The advantages of this approach are that it reduces the complexities associated with emitting file logs (such as parsing, tailing, and rotation), emits logs in a structured format, and allows logs to be sent directly to the logging backend without a log collection agent.
However, this approach is not without its disadvantages. It removes local log files, which simplifies local log reading from the equation. It also adds a compatibility challenge; the logging backend must be capable of receiving logs from OTLP or any other OpenTelemetry-compatible network protocol.
To facilitate the approaches discussed above, OpenTelemetry offers a Bridge API and SDK. These tools can be used alongside existing logging libraries to automatically include trace context in emitted logs and simplify the process of sending logs via OTLP. Log appenders utilize the API to bridge logs from existing libraries to OpenTelemetry’s data model, and the SDK ensures proper processing and export of the logs.
The image above displays how the two approaches work in legacy first-party application logs, third-party application logs, and system logs. The diagram below shows how a new first-party application uses OpenTelemetry API, SDK, and the existing log libraries.
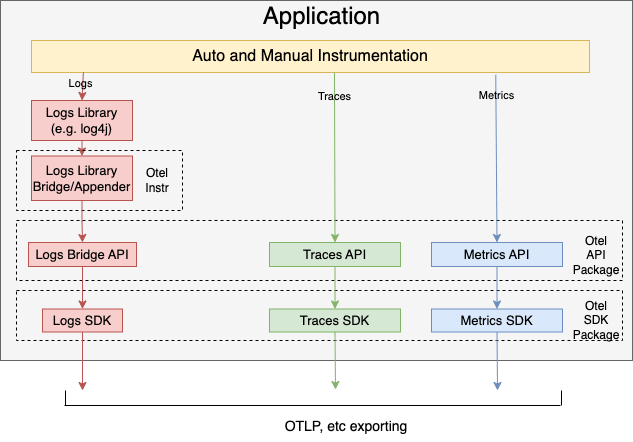
The logs are directed to the OpenTelemetry Collector via OTLP. OpenTelemetry Collector’s logs apparently follow the OTel Logs Data Model, which eases the process.
Having explained the architecture of the OpenTelemetry Data Model, let’s consider why and how OpenTelemetry improves on existing logging solutions.
Limitations of Existing Logging Solutions
Existing logging solutions have facilitated the monitoring and troubleshooting of legacy apps. However, the following limitations render them inadequate for modern apps.
Telemetry Volume
Some logging solutions struggle to handle the high volume of logs generated by modern applications. They often become overwhelmed, leading to performance issues and delays in log processing, which may hamper the observability process.
Integration
Legacy logging solutions either lack proper integrations or require additional configurations to work seamlessly with modern systems. Finding specific log events or patterns and implementing complex querying, filtering and log correlation can be challenging in traditional logging solutions. Oftentimes, it requires additional tools (which may be difficult to integrate) or manual effort.
Data Storage
These logging solutions have limitations on the amount of data they can store or the retention periods they can support. This often results in the loss of historical log data, making it difficult to analyze app events or behavioral trends over time.
Standardization
Logging frameworks, libraries and apps may use diverse log formats that can be problematic for DevOps teams seeking to process logs from various microservices.
Similarly, the lack of standardization means log formats emitted by a legacy solution may not be compatible with a preferred observability solution. Oftentimes, you’d be required to make additional effort to transform and normalize your logs before analysis.
OpenTelemetry helps overcome these limitations with its Logs Data Model and vendor-agnostic backend support. This way, OpenTelemetry handles and eases log collection, standardization and correlation while your chosen backend ensures you can interpret your logs.
It is important to choose your logging solution based on its scalability, storage capabilities, log format support, search and analysis features, real-time monitoring capabilities, and integration with other monitoring systems.
The good news is that our observability framework, Middleware, offers these while tackling the limitations of legacy logging solutions. The platform works seamlessly with OpenTelemetry and its telemetry data models and has a number of features that set it apart from other logging solutions.
- Middleware combines and correlates metrics, logs and traces in one unified platform for root-cause analysis and end-to-end visibility into your applications’ status.
- It is built on a scalable infrastructure that can handle large data ingestion rates and process logs in real-time.
- It offers powerful log processing capabilities, which allow you to perform advanced analytics, filtering, and search queries on your log data.
- Middleware enables you to set up intelligent alerting, where you define thresholds, patterns or specific conditions to trigger alerts when certain log events occur. This ensures proactive notification, reduces alerting fatigue and facilitates swift issue remediation.
Middleware: An OpenTelemetry-Based Full-Stack Observability Platform
Middleware is a comprehensive observability platform which is built to analyze telemetry collected from OTel. It provides log monitoring screens for end-to-end visibility into your applications.
- To get started with Middleware, create an account.
- Once you’re logged in, the Middleware Unified Observability dashboard comes up. The dashboard provides a high-level overview of your system’s health, performance and key metrics.
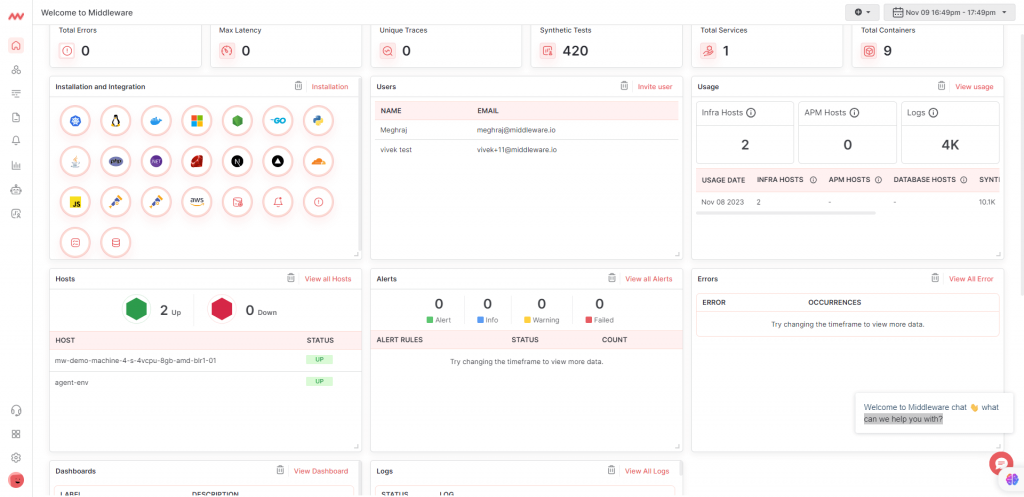
- The screen below shows the many frameworks Middleware seamlessly integrates with, including OpenTelemetry. The OTel icons on the screen are for metrics and logs.
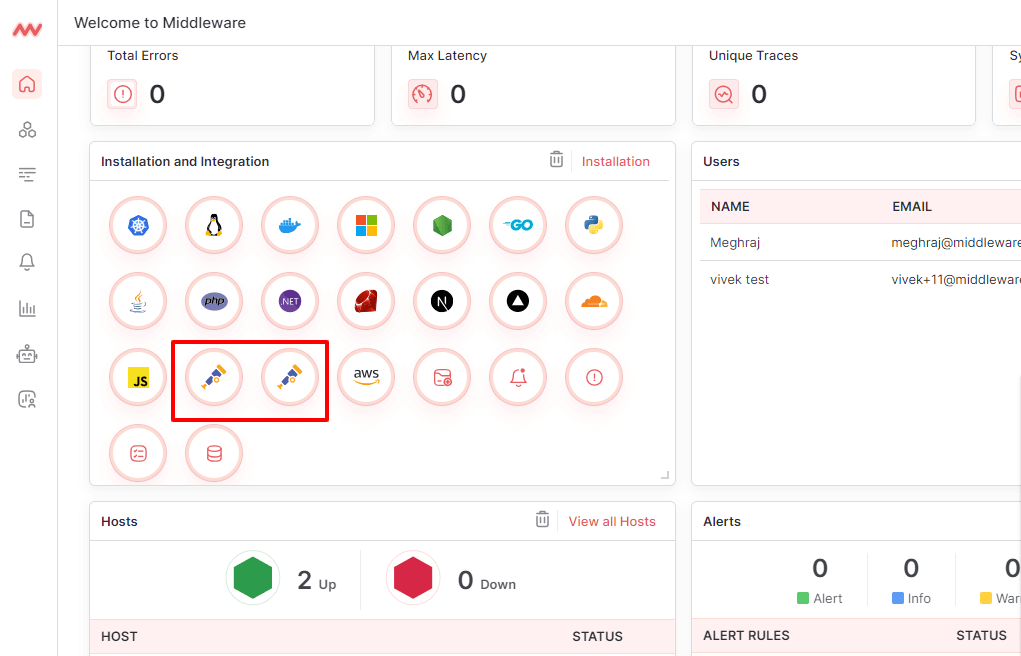
- Middleware offers a dedicated log monitoring section—the icon on the left side of the screen—that allows you to view and analyze your log data in a centralized and intuitive manner.
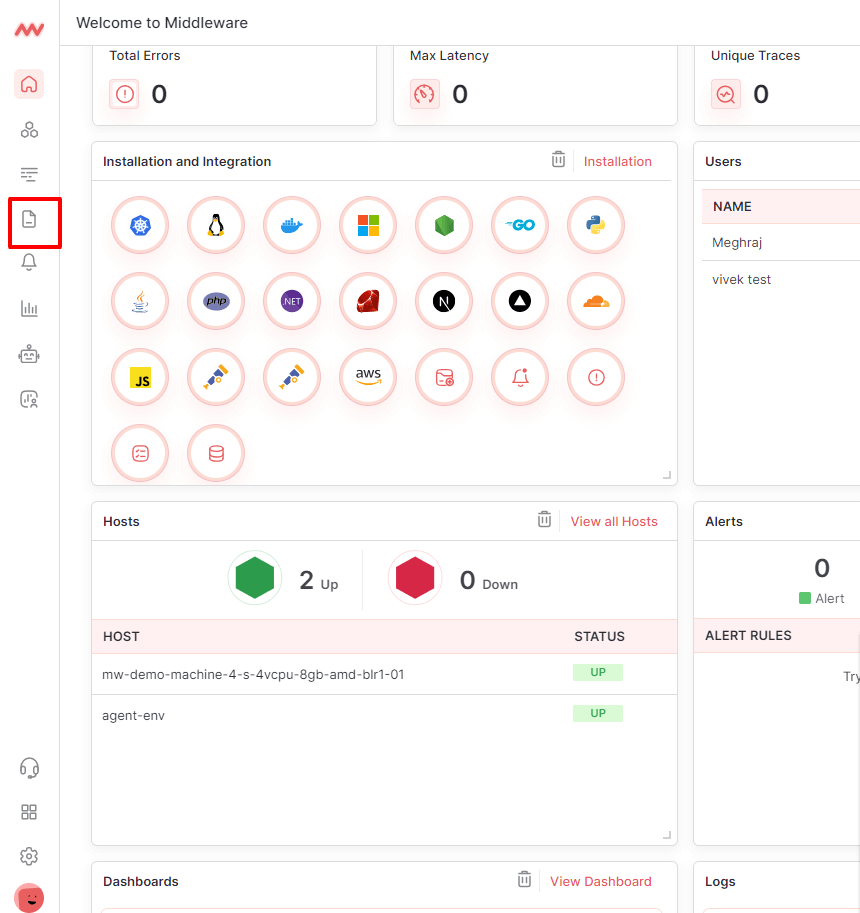
- When you click on the logs icon, you’ll have the screen below.
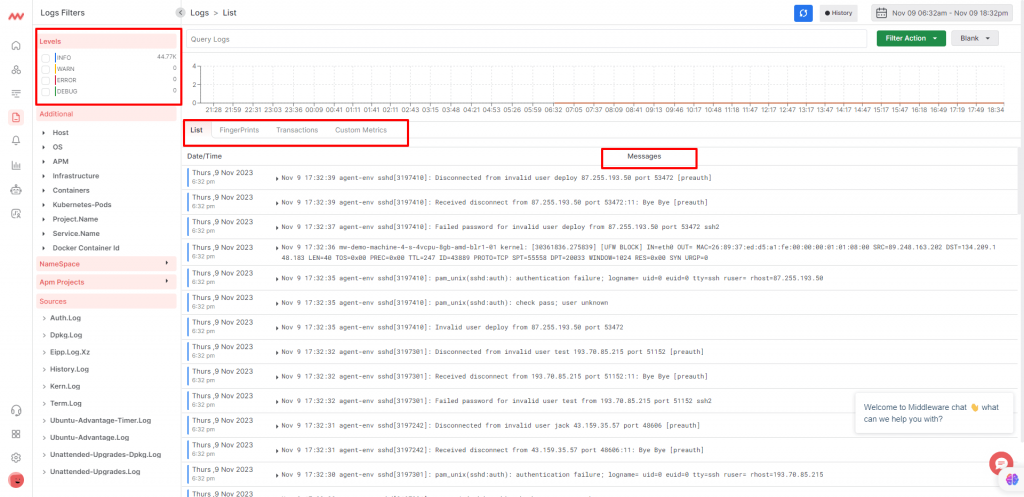
Within this section, you’ll find the log filter options, which enable you to filter log messages based on specific criteria such as timestamp, log level, keywords, or custom attributes. On the upper left are the log levels. When you check the box on each “level,” Middleware will display messages from the selected level.
- You can further analyze your logs by clicking on individual messages. When you click on the first log message, the screen below is what you’ll have.
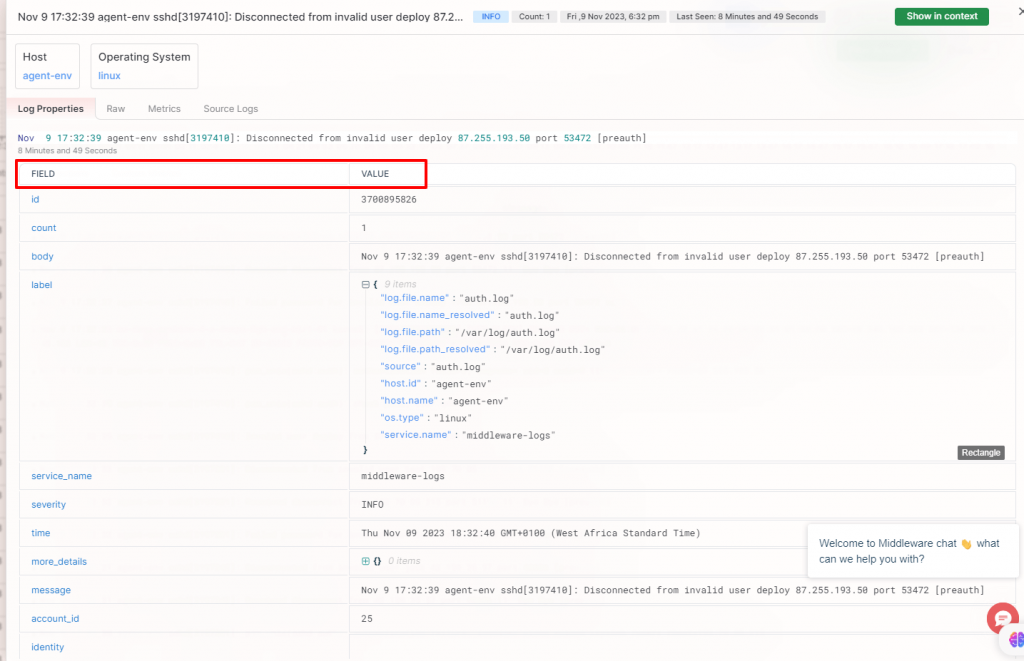
On this screen, you’ll see the log record with the required fields and their values, which include body, timestamp and severity, among others.
- Above the fields, when you click on “Source Logs,” you’ll find a more refined analysis with in-depth timestamps of the events. This section allows you to focus on specific log streams, sources or attributes, enabling you to understand specific log contexts and troubleshoot effectively.
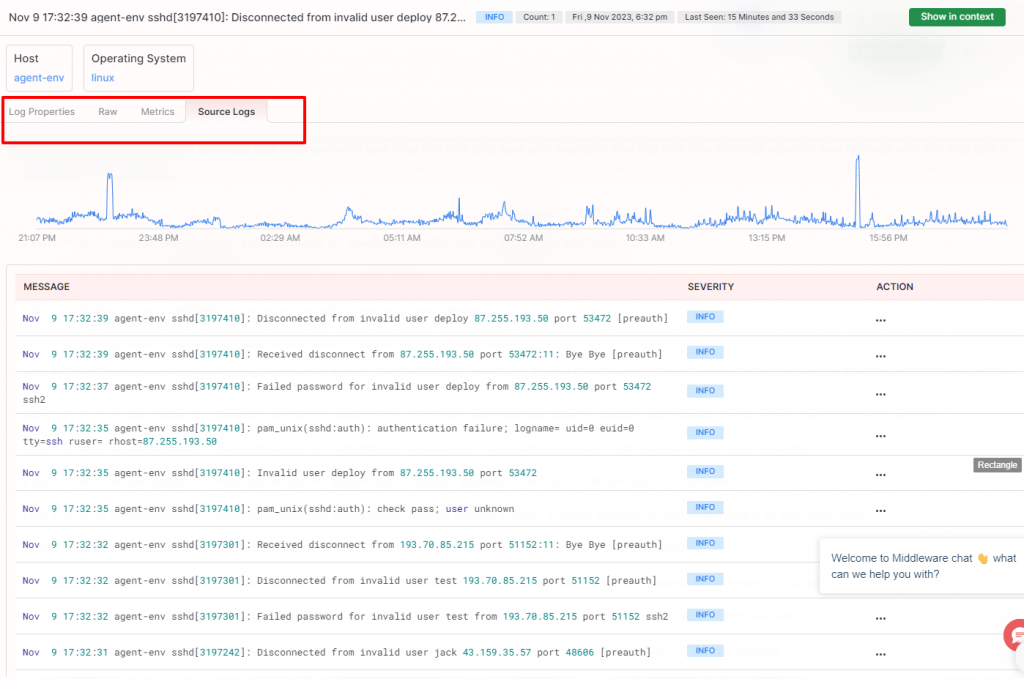
- Finally, on the home screen, when you navigate to “view dashboard” and then “unified dashboard,” you’ll find your logs, metrics, and traces all correlated on a single page. This unified page also allows you to visualize log patterns, trends, and anomalies through interactive charts and graphs.
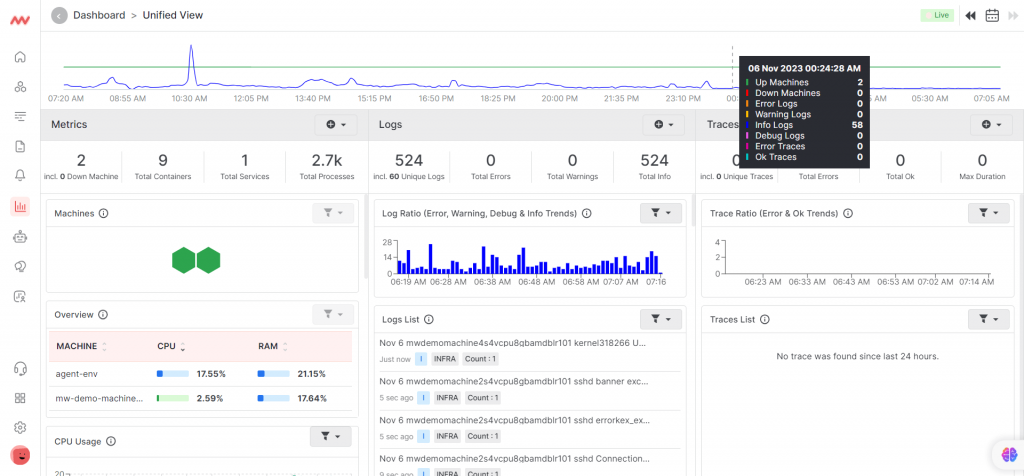
In addition, the platform allows you to set up intelligent log-based alerts, which are sent via various channels such as Slack or Microsoft Teams to notify you when specific log events occur. You can configure alert rules based on log message patterns, log levels, or specific attributes.
By leveraging Middleware’s array of powerful log visualization and analysis functionalities for your OpenTelemetry-instrumented applications, you can proactively identify and resolve issues, resulting in better software quality and user experience.
FAQ
How do you collect logs in OpenTelemetry?
Logs can be collected via File or Stdout Logs, sent directly to the Collector, depending on your app’s requirements and your preferences. Both options have their pros and cons.
What is the difference between Log and Event in OpenTelemetry?
While logs capture discrete events and messages, events capture the context of operations, including timing and causal relationships between different components of an app.
What is the difference between Telemetry and Log?
Telemetry is a broader term that encompasses logs, metrics, and traces, while logs specifically refer to captured messages or events representing the state and execution of an app.