Observability is crucial for any modern organization since we are now more heavily reliant on software and hardware systems than ever before. An estimated 100 zettabytes of data will be stored in the cloud by 2025, with data volumes growing at an average of 63% each month in a company.
With the rising amount of data comes the risk of managing, storing, and analyzing this monumental data. Observability tools help organizations proactively identify and resolve performance issues, optimize resource allocation, and ensure exceptional user experiences. However, building a robust observability stack can sometimes lead to concerns about cost management.
This is a big challenge for most data-driven companies, and it is important to strike a balance between achieving comprehensive visibility and keeping your observability costs in check. This blog dives deep into five practical tips that will help you optimize your observability setup without sacrificing the valuable insights it delivers.
5 tips to reduce your observability costs without sacrificing visibility
As with any IT infrastructure, performance issues will depend on the size and complexity of an activity. Highly intensive processes or large-scale activities can lead to slow load times, lags, or, at times, even outages. This is a major reason why the DevOps teams need to continuously monitor system behavior, utilizing observability pillars like metrics, logs, and traces to ensure that the system is running efficiently and that any spike in activity can be mitigated before it can make a huge impact on the overall system or specific applications.
However, with rising data, organizations today are looking to mitigate observability costs without sacrificing visibility. To do so, here are five key tips to optimize your observability setup and streamline your spending:
1. Optimize your data ingestion
In most organizations, data quality is a process that begins only after the data makes its way into a common storage or warehouse. However, many observability tools charge based on the volume of data ingested, and this can often lead to higher costs for observability. Hence, the first step to cost reduction is identifying and capturing only the data that truly matters for your specific needs.
Here’s how to refine your data ingestion strategy for cost-effectiveness:
- Focus on Critical Metrics: Prioritize capturing metrics that directly impact application performance and user experience. These might include:
- Application Response Times: Identify and track key metrics like server response times, API latencies, and page load times.
- Resource Utilization: Monitor CPU, memory, and network usage across your infrastructure to identify potential bottlenecks.
- Error Rates: Track the occurrence of errors and exceptions within your applications.
- Filter and Whitelist Data Sources: Many observability platforms like Middleware allow you to filter data at the source. Leverage this functionality to exclude irrelevant data like debug logs or information from non-critical services. Whitelist only the specific data sources that provide valuable insights for your monitoring needs.
- Sample Strategically: For high-volume data streams, consider implementing data sampling techniques. This allows you to capture a statistically significant subset of the data, reducing storage and processing costs while still providing valuable insights into overall system behavior. However, be mindful of the sampling rate to ensure you capture enough data to identify anomalies or trends.
- Leverage Data Transformation Rules: Transform raw data into a more compact and efficient format before ingestion. Tools like Logstash can be used to parse logs, extract relevant fields, and remove unnecessary information. This reduces the overall volume of data stored and minimizes processing overhead.
- Utilize Compression Techniques: Some observability platforms offer data compression options. Compressing data before storage can significantly reduce storage requirements and associated costs. With Middleware, you can leverage container monitoring to gain end-to-end visibility into the performance of a containerized environment. This ensures that you gain deeper insights into a specific containerized environment, which can include defined applications, multi-cloud and hybrid environments, and other aspects of a defined container.
2. Lower data retention
After data ingestion comes data retention policies; these play a crucial role in observability costs, as observability often requires logs and traces for analysis. When stored, they provide a historical record of what has been happening in your IT systems, helping you to resolve any troubleshooting errors and gain insights into the system’s healthy behavior. However, storing logs and traces for a long period of time can be expensive and increases both the volume and cost of maintaining this infrastructure.
To reduce these costs and lessen resolution time, organizations can do the following:
- Identify Retention Requirements: Define clear retention periods for different data types. Critical metrics and logs essential for day-to-day operations may require longer retention (e.g., a week or a month). Less critical data, like debug logs or traces from infrequent events, can have shorter retention periods (e.g., a few days).
- Utilize Tiered Storage: Many observability platforms offer tiered storage options. Frequently accessed data can be stored in high-performance but potentially more expensive storage tiers. Meanwhile, less frequently accessed historical data can be archived to cost-effective cold storage solutions.
- Leverage Data Lifecycle Management (DLM): Implement automated data lifecycle management tools to automate data archiving and deletion based on predefined retention policies. This ensures you’re not paying to store data that has outlived its usefulness.
3. Embrace dynamic observability
Traditional, static observability approaches often collect data at a constant rate, regardless of system activity. This “one-size-fits-all” method can be inefficient and costly, especially for applications with fluctuating workloads. Dynamic observability allows you to be more cost-effective, using specialized methods like:
- Scaling Data Collection Based on Need: Dynamic observability tools can automatically adjust the amount of data collected based on real-time system behavior. During periods of high traffic or potential issues, data collection can be increased to provide deeper insights. Conversely, during low-activity periods, data collection can be reduced, minimizing storage and processing overhead.
- Focusing on Anomaly Detection: Leverage AI-powered anomaly detection capabilities within your observability platform. These tools can pinpoint deviations from normal system behavior, allowing you to focus data collection efforts on identifying and resolving potential issues. This targeted approach reduces unnecessary data capture and associated costs.
- Utilizing Ephemeral Tracing: For short-lived tasks or microservices with transient execution, consider using ephemeral tracing techniques. Ephemeral traces are designed for short-term analysis and automatically expire after a predefined period, eliminating the need for long-term storage and associated costs.
4. Consolidate tooling
One of the biggest challenges most organizations face when it comes to observability is maintaining data and real-time monitoring across multiple tools. A typical tech stack in an organization includes multiple apps, systems, and environments.
Unfortunately, this large-scale dependence on specialized tools can lead to what is known as observability tool sprawl, increasing complexity and driving up costs.
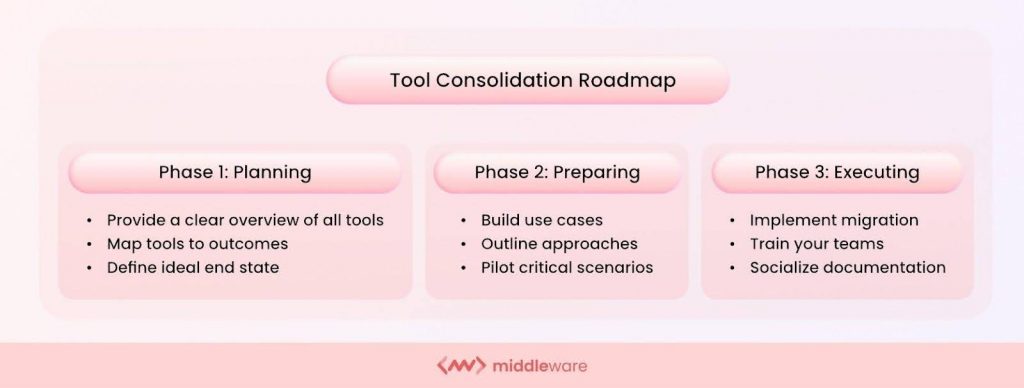
To resolve this, you can leverage the consolidate tooling method, which includes:
Step 1: Consolidate your observability stack
Multiple tools often collect overlapping data sets, leading to unnecessary redundancy and inflated storage costs. Consolidating your observability stack onto a unified platform eliminates duplication and streamlines data management.
Step 2: Simplify tooling into a single platform
Managing a multitude of tools can be time-consuming and resource-intensive. A single platform simplifies configuration, maintenance, and user access, reducing administrative overhead and associated costs.
Step 3: Execute
Once you have evaluated the options, start consolidating your tools to the singular platform by purchasing new software or simplifying internal processes. Consolidation fosters a holistic view of your system’s health. By correlating data from various sources within a single platform, you gain deeper insights into application performance and user experience, allowing for more proactive problem-solving.
5. Choose the right tool for your setup
Not all observability platforms are created equal. Selecting the right tool for your specific needs is crucial for optimizing costs. Some factors you should consider when selecting an observability platform include:
- Deployment model: Choose a tool that aligns with your deployment model (on-premises, cloud, or hybrid). On-premise solutions may require upfront infrastructure costs, while cloud-based options typically offer flexible pricing models based on usage.
- Feature set: Carefully evaluate the features offered by different platforms. Prioritize tools that provide the functionalities you truly need, avoiding feature bloat that can increase costs.
- Scalability: Select a platform that can scale alongside your evolving infrastructure needs. Opt for solutions with flexible pricing tiers that adapt to your data volume and user base, ensuring you only pay for the resources you utilize.
Middleware is a full-stack cloud observability platform that allows businesses to monitor their cloud infrastructure in real-time. It consolidates metrics, logs, traces, and events into a unified timeline. Plus, its core features can be used across multiple environments, including on-prem, cloud, and hybrid deployment options. This ensures comprehensive viability at a cost-effective price point.
Conclusion
Optimizing your observability setup for cost-effectiveness requires a proactive approach. By implementing the strategies outlined above, you can achieve a balance between gaining valuable insights and keeping your observability costs under control.
To get a quick recap of our blog, remember that to keep your costs in control, you should:
- Focus on collecting only the data that truly matters for your specific needs
- Implement data retention policies and leverage tiered storage for cost-efficiency
- Embrace dynamic observability to scale data collection based on system activity
- Consolidate your observability tools to reduce redundancy and streamline management
- Choose the right tool that aligns with your deployment model, feature needs, and scalability requirements
Middleware offers a modern pricing model ideal for your agile approach to help you simplify your observability pricing. It offers three pricing models, which you can choose from depending on your usage and requirements.
For specific features, such as infrastructure monitoring, log monitoring, application monitoring, synthetic testing, database monitoring, serverless monitoring, or real user monitoring, you can add them to your overall pricing model and pay-as-you-go. This ensures you’re not paying for unnecessary features or data storage.
Contact Middleware today to learn more about how our cost-effective observability solutions can empower your teams and optimize your infrastructure performance.